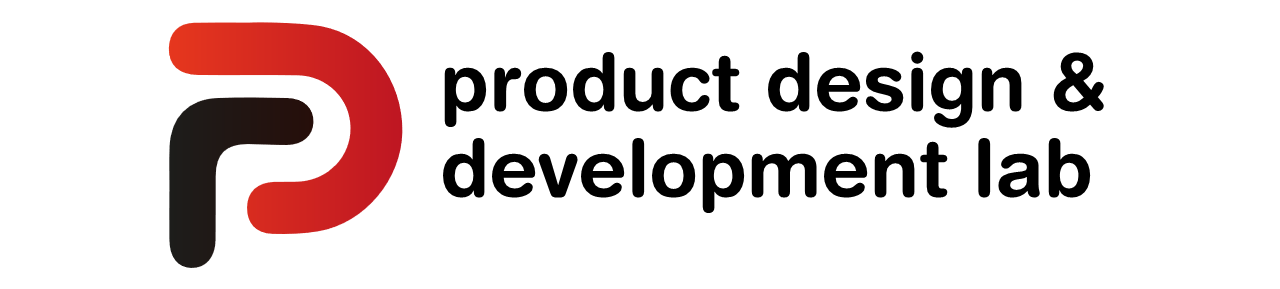
Welcome to the Product Design and Development Lab, led by Prof. Stephen Ekwaro-Osire.
We specialize in risk and uncertainty quantification in engineering systems. With
our expertise in artificial intelligence and digital twin concept, we enable robust
decision-making in complex engineering scenarios.
Join us in shaping the future of product designand development through transformative
technologies.