Simone Scaringi, Ph.D.
Assistant Professor
Department of Physics and Astronomy
Email: simone.scaringi@ttu.edu
Phone: (+1) 806-834-1300
Office: 115 Science Building
Personal Webpage: www.phys.ttu.edu/~simo
Education:
Ph.D., Astrophysics, University of Southampton, 2010
M.Phil. Astrophysics, University of Southampton, 2007
B.Sc. Mathematics with Astronomy, University of Southampton (1st Class Hons), 2005
Awards and Fellowships:
Alexander von Humboldt Fellowship, 2014
FWO Pegasus Marie Curie Fellowship, 2012
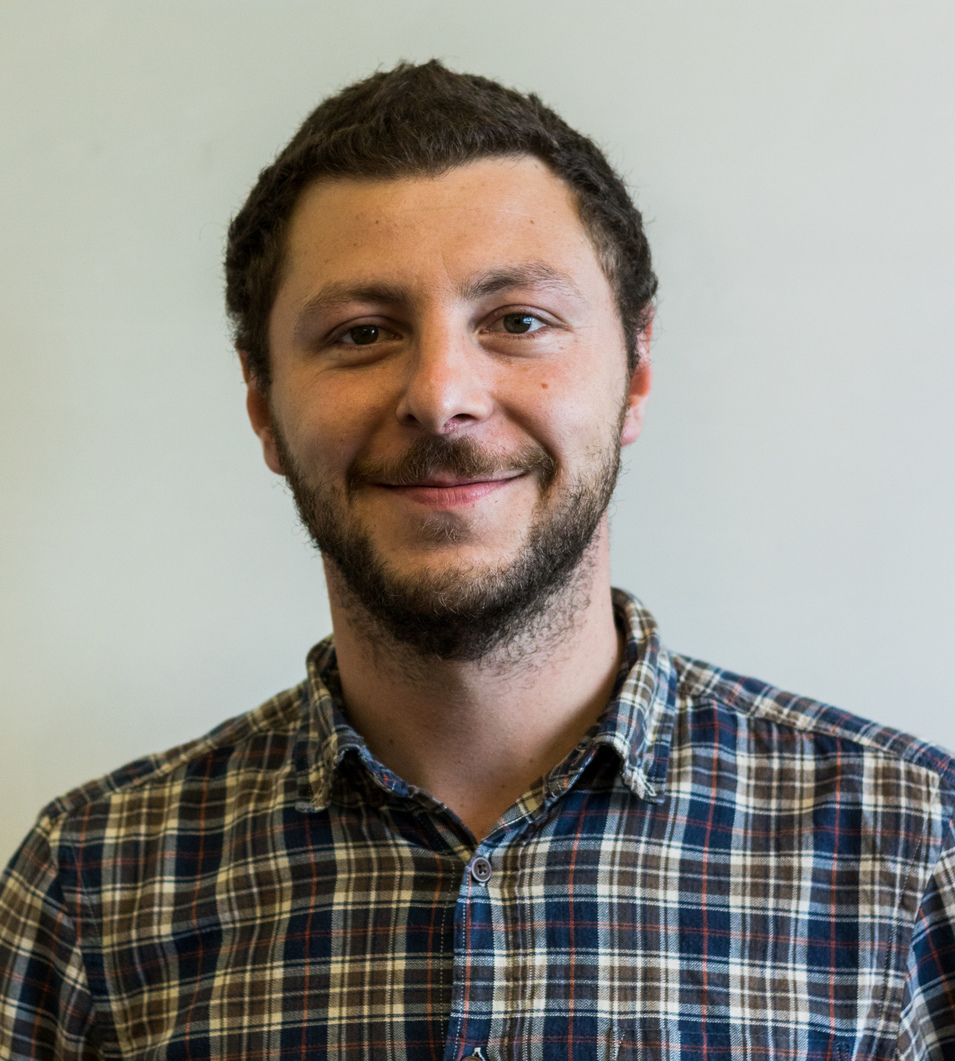
Research Interests
My research is mostly focused on 1) understanding disk accretion on all mass and size scales and 2) the development and application of novel machine learning algorithms to large astrophysical data.Accretion disk physics
Accretion is the process that drives and regulates the growth of most objects in the Universe: from the birth of stars to the feeding of supermassive black holes, from the evolution of galaxies over cosmological time scales to the life cycles of interacting binary stars in Solar neighborhood. My research focus is to test whether the physics of accretion can be thought of as self-invariant and "universal" across the full mass and size scale of different accreting objects. I do this mostly through the use of both ground- and space-based observations across the electromagnetic spectrum.
Machine Learning and BlackGEM
The BlackGEM telescope array, expected to be operational in 2020, is an array of 65cm telescopes dedicated to pinpointing the optical counterparts to gravitational wave events. The first 3 unit telescopes will be placed at La Silla (Chile). The operations of BlackGEM (which include a southern all-sky survey, a fast synoptic survey, as well a deep-drill fields for long term fast photometry) allow for a very wide range of astrophysics research to be performed. This large project is bound to require robust, autonomous, and fast algorithms, to correctly classify over 1 billion southern targets, and promptly identify new optical transients minutes after observations. I am currently exploring the use of novel machine learning algorithms such as Deep Learning in combination with Convolutional Neural Networks in preparation for the incoming BlackGEM data stream. I am particularly interested in removing as much ``subjectivity'' as possible from any classification algorithm, as well as implementing objective data fusion techniques, and Deep Learning appears very promising in this respect.
Selected Publications
- Scaringi, S., Maccarone, T. J., D'Angelo, C., Knigge, C., Groot, P. J. Magnetically gated accretion in an accreting ‘non-magnetic' white dwarf, Nature, Vol. 552, Issue 7684, pp. 210-213 (2017).
- Scaringi, S., Manara, C. F., Barenfeld, S. A., Groot, P. J., Isella, A., Kenworthy, M. A., Knigge, C.; Maccarone, T. J., Ricci, L., Ansdell, M., The peculiar dipping events in the disc-bearing young-stellar object EPIC 204278916, MNRAS, Vol. 463, Issue 2, p.2265-2272 (2016)
- Scaringi, S., Maccarone, T. J., Kording, E., Knigge, C., Vaughan, S., Marsh, T. R., Aranzana, E., Dhillon, V. S., Barros, S. C. C. Accretion-induced variability links young stellar objects, white dwarfs, and black holes, Science Advances, vol. 1, issue 9, pp. e1500686 (2015)
- Scaringi, S.,A physical model for the flickering variability in cataclysmic variables, MNRAS, Vol. 438, Issue 2, p.1233-1241 (2014).
- Scaringi, S., Körding, E., Uttley, P., Knigge, C., Groot, P. J., Still, M. The universal nature of accretion-induced variability: the rms-flux relation in an accreting white dwarf, MNRAS, Vol. 421, Issue 4, pp. 2854-2860 (2012)
- Scaringi, S., Bird, A. J., Norton, A. J., Knigge, C. et al., Hard X-ray properties of magnetic cataclysmic variables, MNRAS, Vol 401, Issue 4, pp. 2207-2218 (2010).
- Knigge, C., Scaringi, S., Goad, M. R., Cottis, C. E., The intrinsic fraction of broad-absorption line quasars, MNRAS, Vol. 386, Issue 3, pp. 1426-1435 (2008)
Department of Physics and Astronomy
-
Address
Texas Tech University, Physics & Astronomy Department, Box 41051, Lubbock, TX 79409-1051 -
Phone
806.742.3767 | Fax: 806.742.1182 -
Email
physics.astronomy.webmasters@ttu.edu | physics.academic.advising@ttu.edu